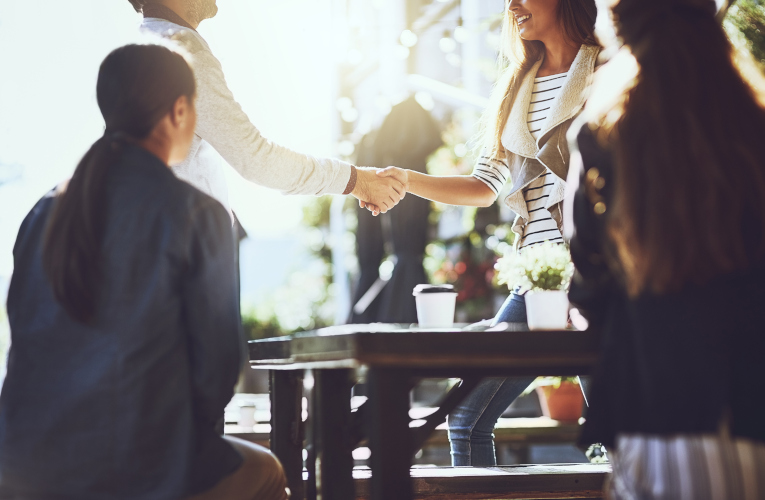
Forecasting
A Tribute to Professor George Dantzig
Throughout my working life, I have been fortunate to have the opportunity to work with brilliant econometricians and mathematicians. Very early in my career, while at my first job after finishing my graduate degree, I was afforded the opportunity to work with Professor George Dantzig. For those of you who are not familiar with Professor Dantzig, I encourage you to read about him here.
Unfortunately, at the time, the internet and Wikipedia did not exist. So, I knew nothing about his history when the opportunity fell in my lap to work with one of the 20th century’s most brilliant mathematicians. I didn’t even know he was a fellow Cal Bear. When I first met Professor Dantzig, we were walking across the Stanford campus from his office to his home, which was located on the edge of campus. I recall students and faculty going out of their way just to say hi to him. In return, they all received the same response: “It is so nice to see you, you look great.” We left a trail of smiling faces. I recall thinking, “who was this professor that made people feel so good?” Since I was an economist and not a mathematician, I had no clue who Professor Dantzig was. I was simply informed that I should work with Professor Dantzig on a new least cost gas dispatch model. At the time, I knew nothing about linear optimization modeling, but under Professor Dantzig’s patient tutelage, I was able to design a next-generation gas optimization model that is still in use today.
This experience changed my perspective on mathematical modeling. As econometricians, we solve unconstrained optimization problems (i.e., linear regression). Professor Dantzig opened my mind to the world of constrained optimization problems and their solutions. The Simplex Algorithm that Professor Dantzig invented provides invaluable insights into crafting an optimization problem that will return a sensible solution. With the Simplex Algorithm, Dantzig exploited the simple fact that most solutions to constrained optimization problems are corner solutions.
The following example will illustrate what I mean by a corner solution. Let there be two unknowns: X and Y. The objective function is to find values for X and Y where Z = X + Y is maximized. Since the objective function Z = X + Y is unconstrainted, we need to place constraints on the possible values of X and Y to avoid the nonsensical solution of Z equals infinity. Let’s assume 0<= X <= 100 and 0 <= Y <= 200. With these constraints, we recast the optimization problem as finding the values of X and Y that maximize Z, all subject to 0<= X <= 100 and 0 <= Y <= 200.
To find the solution, the Simplex Algorithm initializes the solution by starting with the origin. At the origin, X = 0 and Y = 0, giving the objective function value Z = 0 + 0. The Simplex Algorithm then proceeds by letting X be as big as possible, which in this case is X = 100. The new solution Z = 100 + 0 = 100 is greater than the first solution so we move to the corner (X=100, Y=0). Since X cannot get any bigger, the Simplex Algorithm proceeds by letting Y be as big as possible. This gives the corner solution Z = 100 + 200 = 300. Since neither X nor Y can be any bigger, this last corner solution is the optimal solution. If you want a different solution, you either must change the constraints imposed on X and Y, or you must introduce additional constraints that will bind the solution to a value less than 300. The beauty of constrained optimization problems is they will always find corner solutions. To get realistic solutions, it is up to the model designer to craft objective functions and constraints that will produce realistic solutions. Constrained optimization models are truly some of the most fun types of models to build.
That was a lovely story about Professor Dantzig, but why did I write this blog? As it turns out, forecasting Net Loads at the Grid Edge opens the door to a wide range of constrained optimization problems that, if formulated well, can be used to forecast how customers will leverage Battery Energy Storage Systems, electric vehicle (EV) charging patterns and excess solar photovoltaic (PV) generation to minimize their costs or possibly maximize their revenue from wholesale energy trades. For me, that means I am dusting off the work I did with Professor Dantzig. It also means that we, as forecasters, need to learn the language of Network Modelers who design mathematical representations of the High, Medium and Low Voltage Networks. Specifically, we need to understand that solutions to network models will seek corner solutions, like not charging your EV because it minimizes costs or selling all the solar PV electricity instead of consuming power because it will maximize your profits.
To avoid these types of corner solutions, we need to be prepared to provide plausible upper and lower limits on key decision variables, including:
- How much and when to start EV charging
- How much and when to discharge battery storage
- How much power can be sold back to the grid
By combining accurate point forecasts with plausible forecast limits, we can help avoid nonsensical corner solutions, which compound an already complicated network modeling problem. So, the next time you visit the Corner Café on Standford’s campus, say “hi” to those you encounter, because everybody likes to hear “It is so nice to see you; you look great.”
Related Articles
HTML Example
A paragraph is a self-contained unit of a discourse in writing dealing with a particular point or idea. Paragraphs are usually an expected part of formal writing, used to organize longer prose.